In-field hyperspectral imaging: An overview on the ground-based applications in agriculture
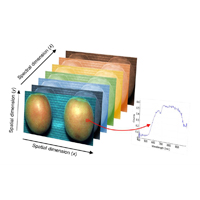
Published: 29 September 2020
Abstract Views: 3351
PDF: 1989
HTML: 1352
HTML: 1352
Publisher's note
All claims expressed in this article are solely those of the authors and do not necessarily represent those of their affiliated organizations, or those of the publisher, the editors and the reviewers. Any product that may be evaluated in this article or claim that may be made by its manufacturer is not guaranteed or endorsed by the publisher.
All claims expressed in this article are solely those of the authors and do not necessarily represent those of their affiliated organizations, or those of the publisher, the editors and the reviewers. Any product that may be evaluated in this article or claim that may be made by its manufacturer is not guaranteed or endorsed by the publisher.
Similar Articles
- Juan Ãngel Mintegui Aguirre, José Carlos Robredo Sánchez, Carlos de Gonzalo Aranoa, Pablo Huelin Rueda, Jorge Fallas, Felipe Cisneros, Pedro Cisneros, Adriana Urciuolo, Rodolfo Iturraspe, Forest use strategies in watershed management and restoration: application to three small mountain watersheds in Latin America , Journal of Agricultural Engineering: Vol. 45 No. 1 (2014)
- Alessandro Trevisani, Eleonora Iaccheri, Angelo Fabbri, Adriano Guarnieri, Pallet standards in agri-food sector: a brief survey , Journal of Agricultural Engineering: Vol. 45 No. 2 (2014)
- Ernest Ekow Abano, Hai Le Ma, Wenjuan Qu, Thin-layer catalytic far-infrared radiation drying and flavour of tomato slices , Journal of Agricultural Engineering: Vol. 45 No. 1 (2014)
- Marco Fiala, Jacopo Bacenetti, Model for the economic, energy and environmental evaluation in biomass productions , Journal of Agricultural Engineering: Vol. 43 No. 1 (2012)
- Ian Torotwa, Qishuo Ding, Emmanuel Awuah, Ruiyin He, Biomimetic tool design improves tillage efficiency, seedbed quality, and straw incorporation during rototilling in conservation farming , Journal of Agricultural Engineering: Vol. 54 No. 1 (2023)
- Fernando Ferreira Abreu, Luiz Henrique Antunes Rodrigues, Monitoring mini-tomatoes growth: A non-destructive machine vision-based alternative , Journal of Agricultural Engineering: Vol. 53 No. 3 (2022)
- Francesco M. Tangorra, Aldo Calcante, Stefano Nava, Gabriele Marchesi, Massimo Lazzari, Design and testing of a GPS/GSM collar prototype to combat cattle rustling , Journal of Agricultural Engineering: Vol. 44 No. 2 (2013)
- Ali Saeed, Alessandro Comegna, Giovanna Dragonetti, Nicola Lamaddalena, Angelo Sommella, Antonio Coppola, Soil electrical conductivity estimated by time domain reflectometry and electromagnetic induction sensors: Accounting for the different sensor observation volumes , Journal of Agricultural Engineering: Vol. 48 No. 4 (2017)
- Francesco Barreca, Giuseppe Cardinali, Carmelo Riccardo Fichera, Luigi Lamberto, Giuseppe Modica, A fuzzy-based model to implement the global safety buildings index assessment for agri-food buildings , Journal of Agricultural Engineering: Vol. 45 No. 1 (2014)
- Dario Friso, Lucia Bortolini, Influence of the trajectory angle and nozzle height from the ground on water distribution radial curve of a sprinkler , Journal of Agricultural Engineering: Vol. 43 No. 1 (2012)
<< < 5 6 7 8 9 10 11 12 13 14 > >>
You may also start an advanced similarity search for this article.