Cropping pattern simulation-optimization model for water use efficiency and economic return
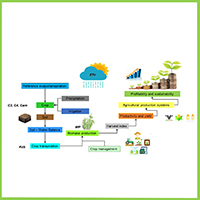
Published:23 December 2021
Abstract Views: 1194
PDF: 527
HTML: 70
HTML: 70
Publisher's note
All claims expressed in this article are solely those of the authors and do not necessarily represent those of their affiliated organizations, or those of the publisher, the editors and the reviewers. Any product that may be evaluated in this article or claim that may be made by its manufacturer is not guaranteed or endorsed by the publisher.
All claims expressed in this article are solely those of the authors and do not necessarily represent those of their affiliated organizations, or those of the publisher, the editors and the reviewers. Any product that may be evaluated in this article or claim that may be made by its manufacturer is not guaranteed or endorsed by the publisher.
Similar Articles
- Abderrahman Sghaier, Hanen Dhaou, Lassaad Jarray , Zouhair Abaab, Ahmed Sekrafi, Mohamed Ouessar , Assessment of drought stress in arid olive groves using HidroMORE model , Journal of Agricultural Engineering: Vol. 53 No. 1 (2022)
- Chiara Antinoro, Vincenzo Bagarello, Vito Ferro, Giuseppe Giordano, Massimo Iovino, Testing the shape-similarity hypothesis between particle-size distribution and water retention for Sicilian soils , Journal of Agricultural Engineering: Vol. 43 No. 3 (2012)
- Mario Pirastru, Marcello Niedda, Mirko Castellini, Effects of maquis clearing on the properties of the soil and on the near-surface hydrological processes in a semi-arid Mediterranean environment , Journal of Agricultural Engineering: Vol. 45 No. 4 (2014)
- Dario Friso, Lucia Bortolini, Influence of the trajectory angle and nozzle height from the ground on water distribution radial curve of a sprinkler , Journal of Agricultural Engineering: Vol. 43 No. 1 (2012)
- Ugo Lazzaro, Caterina Mazzitelli, Benedetto Sica, Paola Di Fiore, Nunzio Romano, Paolo Nasta, On evaluating the hypothesis of shape similarity between soil particle-size distribution and water retention function , Journal of Agricultural Engineering: Vol. 54 No. 4 (2023)
- Ameneh Sobhani, Shawkat B.M. Hassan, Giovanna Dragonetti, Raffaella Balestrini, Mauro Centritto, Antonio Coppola, Alessandro Comegna, Comparing actual transpiration fluxes as measured at leaf-scale and calculated by a physically based agro-hydrological model , Journal of Agricultural Engineering: Vol. 54 No. 3 (2023)
- Luisa Martelloni, Christian Frasconi, Mino Sportelli, Marco Fontanelli, Michele Raffaelli, Andrea Peruzzi, Hot foam and hot water for weed control: A comparison , Journal of Agricultural Engineering: Vol. 52 No. 3 (2021)
- Juan Ãngel Mintegui Aguirre, José Carlos Robredo Sánchez, Carlos de Gonzalo Aranoa, Pablo Huelin Rueda, Jorge Fallas, Felipe Cisneros, Pedro Cisneros, Adriana Urciuolo, Rodolfo Iturraspe, Forest use strategies in watershed management and restoration: application to three small mountain watersheds in Latin America , Journal of Agricultural Engineering: Vol. 45 No. 1 (2014)
- Martine Nyeko, Guido D'Urso, Walter W. Immerzeel, Adaptive simulation of the impact of changes in land use on water resources in the lower Aswa basin , Journal of Agricultural Engineering: Vol. 43 No. 4 (2012)
- Andrea Setti, Giulio Castelli, Lorenzo Villani, Roberto Ferrise, Elena Bresci, Modelling the impacts of water harvesting and climate change on rainfed maize yields in Senegal , Journal of Agricultural Engineering: Vol. 54 No. 3 (2023)
You may also start an advanced similarity search for this article.