Hyperspectral imaging to measure apricot attributes during storage
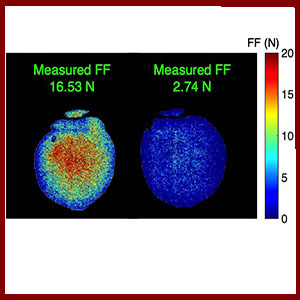
Published: 28 June 2022
Abstract Views: 1000
PDF: 434
HTML: 46
HTML: 46
Publisher's note
All claims expressed in this article are solely those of the authors and do not necessarily represent those of their affiliated organizations, or those of the publisher, the editors and the reviewers. Any product that may be evaluated in this article or claim that may be made by its manufacturer is not guaranteed or endorsed by the publisher.
All claims expressed in this article are solely those of the authors and do not necessarily represent those of their affiliated organizations, or those of the publisher, the editors and the reviewers. Any product that may be evaluated in this article or claim that may be made by its manufacturer is not guaranteed or endorsed by the publisher.
Similar Articles
- Igor Kovacev, Daniele De Wrachien, Report on the 45th International Symposium: Actual Tasks on Agricultural Engineering, 21st-24th February 2017, Opatija, Croatia , Journal of Agricultural Engineering: Vol. 48 No. 2 (2017)
- Fabiana Convertino, Ileana Blanco, Evelia Schettini, Giuliano Vox, A nature-based system for improving Mediterranean buildings’ performance: contribution to energy saving by heat transfer reduction and influence of climatic parameters , Journal of Agricultural Engineering: Vol. 54 No. 3 (2023)
- Shanwen Zhang, Yongyuan Sun, Su Lu, Li Wang, Sian Liu, Zhongliu Wang, Min Dai, Jicheng Gao, Hong Miao, Design and experiment of brush-roller ginkgo leaf picker for the dwarf dense planting mode , Journal of Agricultural Engineering: Vol. 54 No. 4 (2023)
You may also start an advanced similarity search for this article.