E-nose: a low-cost fruit ripeness monitoring system
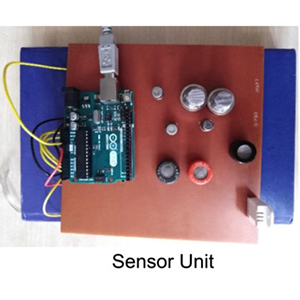
Published: 3 November 2022
Abstract Views: 3054
PDF: 1029
HTML: 755
HTML: 755
Publisher's note
All claims expressed in this article are solely those of the authors and do not necessarily represent those of their affiliated organizations, or those of the publisher, the editors and the reviewers. Any product that may be evaluated in this article or claim that may be made by its manufacturer is not guaranteed or endorsed by the publisher.
All claims expressed in this article are solely those of the authors and do not necessarily represent those of their affiliated organizations, or those of the publisher, the editors and the reviewers. Any product that may be evaluated in this article or claim that may be made by its manufacturer is not guaranteed or endorsed by the publisher.
Similar Articles
- Martin Thalheimer, A low-cost electronic tensiometer system for continuous monitoring of soil water potential , Journal of Agricultural Engineering: Vol. 44 No. 3 (2013)
- Behrooz Behboodi, Mohammad Gholami Parashkoohi, Davood Mohammad Zamani, Saeed Firouzi, A study comparing energy consumption and environmental emissions in ostrich meat and egg production , Journal of Agricultural Engineering: Vol. 56 No. 1 (2025)
- Diwei Wu, Shaohua Zeng, Shuai Wang, Yanan Chen, Yidan Xu, Zanthoxylum infructescence detection based on adaptive density clustering , Journal of Agricultural Engineering: Vol. 55 No. 2 (2024)
- Valentina Giovenzana, Roberto Beghi, Riccardo Guidetti, Massimiliano Luison, Tiziana Nardi, Evaluation of energy savings in white winemaking: impact of temperature management combined with specific yeasts choice on required heat dissipation during industrial-scale fermentation , Journal of Agricultural Engineering: Vol. 54 No. 3 (2023)
- Fabrizio Mazzetto, Pasqualina Sacco, Aldo Calcante, Algorithms for the interpretation of continuous measurement of the slurry level in storage tanks , Journal of Agricultural Engineering: Vol. 43 No. 1 (2012)
- Asiwan Kultongkham, Supakit Kumnon, Tawan Thintawornkul, Teeranoot Chanthasopeephan, The design of a force feedback soft gripper for tomato harvesting , Journal of Agricultural Engineering: Vol. 52 No. 1 (2021)
- Raffaele Cavalli, Stefano Grigolato, Andrea Sgarbossa, Productivity and quality performance of an innovative firewood processor , Journal of Agricultural Engineering: Vol. 45 No. 1 (2014)
- Francesca Piazzolla, Maria Luisa Amodio, Giancarlo Colelli, Spectra evolution over on-vine holding of Italia table grapes: prediction of maturity and discrimination for harvest times using a Vis-NIR hyperspectral device , Journal of Agricultural Engineering: Vol. 48 No. 2 (2017)
- Pietro Catania, Filippa Bono, Claudio De Pasquale, Mariangela Vallone, Closed tank pneumatic press application to improve Sauvignon Blanc wine quality and nutraceutical properties , Journal of Agricultural Engineering: Vol. 50 No. 4 (2019)
- Jiangui Zhao, Tingyu Zhu, Zhichao Qiu, Tao Li, Guoliang Wang, Zhiwei Li, Huiling Du, Hyperspectral prediction of pigment content in tomato leaves based on logistic-optimized sparrow search algorithm and back propagation neural network , Journal of Agricultural Engineering: Vol. 54 No. 4 (2023)
<< < 2 3 4 5 6 7 8 9 10 11 > >>
You may also start an advanced similarity search for this article.