Anthocyanins estimation in homogeneous bean landrace (Phaseolus vulgaris L.) using probabilistic representation and convolutional neural networks
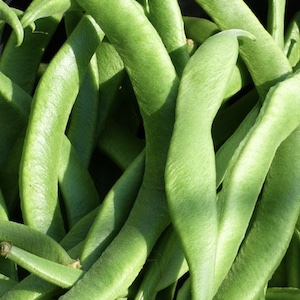
Published: 1 August 2023
Abstract Views: 640
PDF: 277
HTML: 7
HTML: 7
Publisher's note
All claims expressed in this article are solely those of the authors and do not necessarily represent those of their affiliated organizations, or those of the publisher, the editors and the reviewers. Any product that may be evaluated in this article or claim that may be made by its manufacturer is not guaranteed or endorsed by the publisher.
All claims expressed in this article are solely those of the authors and do not necessarily represent those of their affiliated organizations, or those of the publisher, the editors and the reviewers. Any product that may be evaluated in this article or claim that may be made by its manufacturer is not guaranteed or endorsed by the publisher.
Similar Articles
- Simona M.C. Porto, Giulia Castagnolo, Francesca Valenti, Giovanni Cascone, Kernel density estimation analyses based on a low power-global positioning system for monitoring environmental issues of grazing cattle , Journal of Agricultural Engineering: Vol. 53 No. 2 (2022)
- Bhola Paudel, Jayanta Kumar Basak, Seong Woo Jeon, Nibas Chandra Deb, Sijan Karki, Hyeon Tae Kim, Development and field testing of biodegradable seedling plug-tray cutting mechanism for automated vegetable transplanter , Journal of Agricultural Engineering: Vol. 55 No. 2 (2024)
- Giulio Senes, Natalia Fumagalli, Paolo Stefano Ferrario, Daniele Gariboldi, Roberto Rovelli, Municipal community gardens in the metropolitan area of Milano. Assessment and planning criteria , Journal of Agricultural Engineering: Vol. 47 No. 2 (2016)
- Sergio Castellano, Pietro Santamaria, Francesco Serio, Solar radiation distribution inside a monospan greenhouse with the roof entirely covered by photovoltaic panels , Journal of Agricultural Engineering: Vol. 47 No. 1 (2016)
- Bhola Paudel, Jayanta Kumar Basak, Seong Woo Jeon, Gun Ho Lee, Nibas Chandra Deb, Sijan Karki, Hyeon Tae Kim, Working speed optimization of the fully automated vegetable seedling transplanter , Journal of Agricultural Engineering: Vol. 55 No. 2 (2024)
- Adilkhan Niyazbayev, Francesco Garbati Pegna, Kanat Khazimov, Erik Umbetov, Kulmuhanbet Akhmetov, Zhadyra Sagyndykova, Marat Khazimov, Power need of an implement for removing polymer residues from the soil surface in Kazakh horticulture , Journal of Agricultural Engineering: Vol. 53 No. 3 (2022)
- Giacomo Costagli, Matteo Betti, Avocado oil extraction processes: method for cold-pressed high-quality edible oil production versus traditional production , Journal of Agricultural Engineering: Vol. 46 No. 3 (2015)
- Fang Huimin, Niu Mengmeng, Zhu Zhengbo, Zhang Qingyi, Experimental and numerical investigations of the impacts of separating board and anti-blocking mechanism on maize seeding , Journal of Agricultural Engineering: Vol. 53 No. 1 (2022)
- Xin Yu, Ling Zhao, Zongbin Liu, Yiqing Zhang, Distinguishing tea stalks of Wuyuan green tea using hyperspectral imaging analysis and convolutional neural network , Journal of Agricultural Engineering: Vol. 55 No. 2 (2024)
- Nathalie Almaru Caraballo-Arias, Vito Ferro, Assessing, measuring and modelling erosion in calanchi areas: a review , Journal of Agricultural Engineering: Vol. 47 No. 4 (2016)
<< < 8 9 10 11 12 13 14 15 16 17 > >>
You may also start an advanced similarity search for this article.