Lightweight sandy vegetation object detection algorithm based on attention mechanism
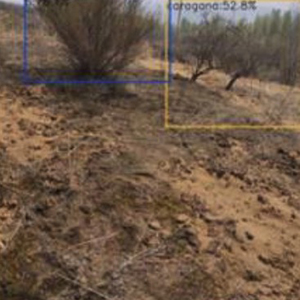
Published:21 November 2022
Abstract Views: 1197
PDF: 293
HTML: 24
HTML: 24
Publisher's note
All claims expressed in this article are solely those of the authors and do not necessarily represent those of their affiliated organizations, or those of the publisher, the editors and the reviewers. Any product that may be evaluated in this article or claim that may be made by its manufacturer is not guaranteed or endorsed by the publisher.
All claims expressed in this article are solely those of the authors and do not necessarily represent those of their affiliated organizations, or those of the publisher, the editors and the reviewers. Any product that may be evaluated in this article or claim that may be made by its manufacturer is not guaranteed or endorsed by the publisher.
Similar Articles
- Kamalesh Kanna S, Kumaraperumal Ramalingam, Pazhanivelan P, Jagadeeswaran R, Prabu P.C., YOLO deep learning algorithm for object detection in agriculture: a review , Journal of Agricultural Engineering: Vol. 55 No. 4 (2024)
- Hongbo Wang, Zhicheng Xie, Yongzheng Yang, Junmao Li, Zilu Huang, Zhihong Yu, Fast identification of tomatoes in natural environments by improved YOLOv5s , Journal of Agricultural Engineering: Vol. 55 No. 3 (2024)
- Xiwang Du, Xia Li, Fangtao Duan, Jiawei Hua, Mengchao Hu, Static laser weeding system based on improved YOLOv8 and image fusion , Journal of Agricultural Engineering: Vol. 55 No. 4 (2024)
- Meng Junjie, Cao Ziang, Guo Dandan, Wang Yuwei, Zhang Dashan, Liu Bingyou, Hou Wenhui, Grape detection in natural environment based on improved YOLOv8 network , Journal of Agricultural Engineering: Vol. 55 No. 4 (2024)
- Maria Elena Menconi, David Grohmann, Statistical assessment of vegetation dynamics within protected areas using remote sensing data , Journal of Agricultural Engineering: Vol. 44 No. 1 (2013)
- Wei Wang, Yuanjuan Gong, Xuewei Bai, Rui Tan, Real-time straw moisture content detection system for mobile straw granulator , Journal of Agricultural Engineering: Vol. 55 No. 2 (2024)
- Jingtao Li, Hao Chen, Guisong Li, Yueqi Liu, Yanli Yang, Xia Liu, Chang Yi Wang, Detection method of potato leaf disease based on YOLOv5s , Journal of Agricultural Engineering: Vol. 55 No. 3 (2024)
- Thanaporn Singhpoo, Khwantri Saengprachatanarug, Seree Wongpichet, Jetsada Posom, Kanda Runapongsa Saikaew, Cassava stalk detection for a cassava harvesting robot based on YOLO v4 and Mask R-CNN , Journal of Agricultural Engineering: Vol. 54 No. 2 (2023)
- Diwei Wu, Shaohua Zeng, Shuai Wang, Yanan Chen, Yidan Xu, Zanthoxylum infructescence detection based on adaptive density clustering , Journal of Agricultural Engineering: Vol. 55 No. 2 (2024)
- Xu Xiao, Yaonan Wang, Yiming Jiang, Haotian Wu, Zhe Zhang, Rujing Wang, AC-YOLO: citrus detection in the natural environment of orchards , Journal of Agricultural Engineering: Vol. 55 No. 4 (2024)
You may also start an advanced similarity search for this article.