3D numerical modelling of temperature and humidity index distribution in livestock structures: a cattle-barn case study
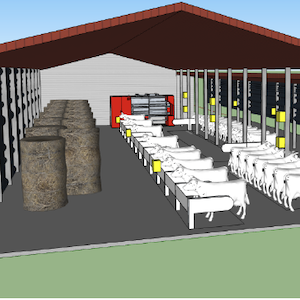
All claims expressed in this article are solely those of the authors and do not necessarily represent those of their affiliated organizations, or those of the publisher, the editors and the reviewers. Any product that may be evaluated in this article or claim that may be made by its manufacturer is not guaranteed or endorsed by the publisher.
Authors
In dairy cattle farming, heat stress largely impairs production, health, and animal welfare. This study aims to develop a workflow and a numerical analysis procedure to provide a real-time 3D distribution of the temperature and humidity index (THI) in a generic cattle barn based on temperature and humidity monitored in sample points, besides characterising the relationship between indoor THI and outside weather conditions. This research was carried out with reference to the study case of a cattle barn. A model has been developed to define the indoor three-dimensional spatial distribution of the Temperature-Humidity Index of a cattle barn based on environmental measurements at different heights of the building. As a core of the model, the Discrete Sibson Interpolation method was used to render a point cloud representing the THI values in the non-sampled areas. The area between 1-2 meters was emphasised as the region of most significant interest to quantify the heat waves perceived by dairy cows. The model represents an effective tool to distinguish different areas of the animal-occupied zone characterised by different values of THI.
How to Cite

This work is licensed under a Creative Commons Attribution-NonCommercial 4.0 International License.