Unmanned aerial vehicle and proximal sensing of vegetation indices in olive tree (Olea europaea)
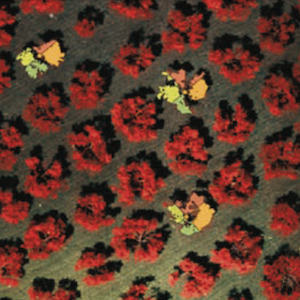
Published: 12 October 2023
Abstract Views: 987
PDF: 434
HTML: 42
HTML: 42
Publisher's note
All claims expressed in this article are solely those of the authors and do not necessarily represent those of their affiliated organizations, or those of the publisher, the editors and the reviewers. Any product that may be evaluated in this article or claim that may be made by its manufacturer is not guaranteed or endorsed by the publisher.
All claims expressed in this article are solely those of the authors and do not necessarily represent those of their affiliated organizations, or those of the publisher, the editors and the reviewers. Any product that may be evaluated in this article or claim that may be made by its manufacturer is not guaranteed or endorsed by the publisher.
Similar Articles
- Dina Statuto, Giuseppe Cillis, Pietro Picuno, Analysis of the effects of agricultural land use change on rural environment and landscape through historical cartography and GIS tools , Journal of Agricultural Engineering: Vol. 47 No. 1 (2016)
- Lorenzo Picco, Alessia Tonon, Riccardo Rainato, Mario Aristide Lenzi, Bank erosion and large wood recruitment along a gravel bed river , Journal of Agricultural Engineering: Vol. 47 No. 2 (2016)
- Zhongkuan Wang, Sheng Wen, Yubin Lan, Yue Liu, Yingying Dong, Variable-rate spray system for unmanned aerial applications using lag compensation algorithm and pulse width modulation spray technology , Journal of Agricultural Engineering: Vol. 55 No. 1 (2024)
- Emanuele Cerruto, INFLUENCE OFAIRFLOW RATE AND FORWARD SPEED ON THE SPRAY DEPOSIT IN VINEYARDS , Journal of Agricultural Engineering: Vol. 38 No. 1 (2007)
- Marco Vieri, Riccardo Lisci, Marco Rimediotti, Daniele Sarri, The RHEA-project robot for tree crops pesticide application , Journal of Agricultural Engineering: Vol. 44 No. s2 (2013): Proceedings of the 10th Conference of the Italian Society of Agricultural Engineering
- Federico Preti, Tommaso Letterio, Shallow landslide susceptibility assessment in a data-poor region of Guatemala (Comitancillo municipality) , Journal of Agricultural Engineering: Vol. 46 No. 3 (2015)
- Sergio Castellano, Pietro Santamaria, Francesco Serio, Solar radiation distribution inside a monospan greenhouse with the roof entirely covered by photovoltaic panels , Journal of Agricultural Engineering: Vol. 47 No. 1 (2016)
- Timothy Denen Akpenpuun, Qazeem Opeyemi Ogunlowo, Wook-Ho Na, Prabhat Dutta, Anis Rabiu, Misbaudeen Aderemi Adesanya, Mohammadreza Nariman, Ezatullah Zakir, Hyeon Tae Kim, Hyun-Woo Lee, Dynamic neural network modeling of thermal environments of two adjacent single-span greenhouses with different thermal curtain positions , Journal of Agricultural Engineering: Vol. 55 No. 2 (2024)
- Marco Grella, Marco Manzone, Fabrizio Gioelli, Paolo Balsari, Harvesting orchard pruning residues in southern Piedmont: a first evaluation of biomass production and harvest loss , Journal of Agricultural Engineering: Vol. 44 No. 3 (2013)
- Francesca Piazzolla, Maria Luisa Amodio, Giancarlo Colelli, The use of hyperspectral imaging in the visible and near infrared region to discriminate between table grapes harvested at different times , Journal of Agricultural Engineering: Vol. 44 No. 2 (2013)
You may also start an advanced similarity search for this article.