Intelligent system based on a satellite image detection algorithm and a fuzzy model for evaluating sugarcane crop quality by predicting uncertain climatic parameters
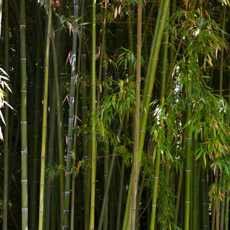
Published: 9 July 2024
Abstract Views: 269
PDF: 59
HTML: 5
HTML: 5
Publisher's note
All claims expressed in this article are solely those of the authors and do not necessarily represent those of their affiliated organizations, or those of the publisher, the editors and the reviewers. Any product that may be evaluated in this article or claim that may be made by its manufacturer is not guaranteed or endorsed by the publisher.
All claims expressed in this article are solely those of the authors and do not necessarily represent those of their affiliated organizations, or those of the publisher, the editors and the reviewers. Any product that may be evaluated in this article or claim that may be made by its manufacturer is not guaranteed or endorsed by the publisher.
Similar Articles
- Andrea Peruzzi, Luisa Martelloni, Christian Frasconi, Marco Fontanelli, Michel Pirchio, Michele Raffaelli, Machines for non-chemical intra-row weed control in narrow and wide-row crops: a review , Journal of Agricultural Engineering: Vol. 48 No. 2 (2017)
- Valentina Giovenzana, Stefano Baroffio, Roberto Beghi, Andrea Casson, Alessia Pampuri, Alessio Tugnolo, Diego De Filippi, Riccardo Guidetti, Technological innovation in the winery addressing oenology 4.0: testing of an automated system for the alcoholic fermentation management , Journal of Agricultural Engineering: Vol. 52 No. 4 (2021)
- Zhongkuan Wang, Sheng Wen, Yubin Lan, Yue Liu, Yingying Dong, Variable-rate spray system for unmanned aerial applications using lag compensation algorithm and pulse width modulation spray technology , Journal of Agricultural Engineering: Vol. 55 No. 1 (2024)
- Yusuf Uzun, Mehmet Resit Tolun, Halil Tanyer Eyyuboglu, Filiz Sarı, An intelligent system for detecting Mediterranean fruit fly [Medfly; Ceratitis capitata (Wiedemann)] , Journal of Agricultural Engineering: Vol. 53 No. 3 (2022)
- Alessandro D'Emilio, Simona M.C. Porto, Giovanni Cascone, Marco Bella, Marco Gulino, Mitigating heat stress of dairy cows bred in a free-stall barn by sprinkler systems coupled with forced ventilation , Journal of Agricultural Engineering: Vol. 48 No. 4 (2017)
- Bing Li, Jiyun Li, Key technology of crop precision sowing based on the vision principle , Journal of Agricultural Engineering: Vol. 54 No. 1 (2023)
- Giuliano Vox, Pierfrancesco Losito, Fabio Valente, Rinaldo Consoletti, Giacomo Scarascia-Mugnozza, Evelia Schettini, Cristoforo Marzocca, Francesco Corsi, A wireless telecommunications network for real-time monitoring of greenhouse microclimate , Journal of Agricultural Engineering: Vol. 45 No. 2 (2014)
- Fuhao Zhu, Jin Chen, Zhuohuai Guan, Yahui Zhu, Hao Shi, Kai Cheng, Development of a combined harvester navigation control system based on visual simultaneous localization and mapping-inertial guidance fusion , Journal of Agricultural Engineering: Vol. 55 No. 3 (2024)
- Ildar Badretdinov, Salavat Mudarisov, Eduard Khasanov, Ruslan Nasyrov, Marat Tuktarov, Operation technological process research in the cleaning system of the grain combine , Journal of Agricultural Engineering: Vol. 52 No. 2 (2021)
- Marzia Quattrone, Giovanna Tomaselli, Lara Riguccio, Patrizia Russo, Assessment of the territorial suitability for the creation of the greenways networks: Methodological application in the Sicilian landscape context , Journal of Agricultural Engineering: Vol. 48 No. 4 (2017)
You may also start an advanced similarity search for this article.