Fine-grained recognition algorithm of crop pests based on cross-layer bilinear aggregation and multi-task learning
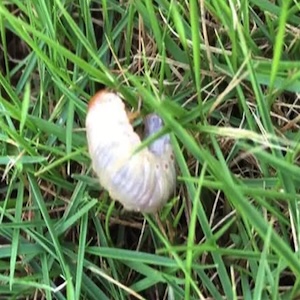
Published:30 October 2024
Abstract Views: 209
PDF: 136
HTML: 1
HTML: 1
Publisher's note
All claims expressed in this article are solely those of the authors and do not necessarily represent those of their affiliated organizations, or those of the publisher, the editors and the reviewers. Any product that may be evaluated in this article or claim that may be made by its manufacturer is not guaranteed or endorsed by the publisher.
All claims expressed in this article are solely those of the authors and do not necessarily represent those of their affiliated organizations, or those of the publisher, the editors and the reviewers. Any product that may be evaluated in this article or claim that may be made by its manufacturer is not guaranteed or endorsed by the publisher.
Similar Articles
- Yiming Xiao, Jianhua Wang, Hongyi Xiong, Fangjun Xiao, Renhuan Huang, Licong Hong, Bofei Wu, Jinfeng Zhou, Yongbin Long, Yubin Lan, Lychee cultivar fine-grained image classification method based on improved ResNet-34 residual network , Journal of Agricultural Engineering: Vol. 55 No. 3 (2024)
- Andrea De Montis, Amedeo Ganciu, Fabio Recanatesi, Antonio Ledda, Vittorio Serra, Mario Barra, Stefano De Montis, The scientific production of Italian agricultural engineers: a bibliometric network analysis concerning the scientific sector AGR/10 Rural buildings and agro-forestry territory , Journal of Agricultural Engineering: Vol. 48 No. s1 (2017): Special Issue
- Marzia Quattrone, Giovanna Tomaselli, Lara Riguccio, Patrizia Russo, Assessment of the territorial suitability for the creation of the greenways networks: Methodological application in the Sicilian landscape context , Journal of Agricultural Engineering: Vol. 48 No. 4 (2017)
- Meng Junjie, Cao Ziang, Guo Dandan, Wang Yuwei, Zhang Dashan, Liu Bingyou, Hou Wenhui, Grape detection in natural environment based on improved YOLOv8 network , Journal of Agricultural Engineering: Vol. 55 No. 4 (2024)
- Xiong Bi, Hongchun Wang, Double-branch deep convolutional neural network-based rice leaf diseases recognition and classification , Journal of Agricultural Engineering: Vol. 55 No. 1 (2024)
- Changjian Zhou, Zhenyuan Zhao, Wenzhuo Chen, Yuquan Feng, Jia Song, Wensheng Xiang, Residual attention based multi-label learning for apple leaf disease identification , Journal of Agricultural Engineering: Vol. 55 No. 4 (2024)
- Xu Xiao, Yaonan Wang, Yiming Jiang, Haotian Wu, Zhe Zhang, Rujing Wang, AC-YOLO: citrus detection in the natural environment of orchards , Journal of Agricultural Engineering: Vol. 55 No. 4 (2024)
- Kai Tian, Jiefeng Zeng, Tianci Song, Zhuliu Li, Asenso Evans, Jiuhao Li, Tomato leaf diseases recognition based on deep convolutional neural networks , Journal of Agricultural Engineering: Vol. 54 No. 1 (2023)
- Francisco Ayuga, Present and future of the numerical methods in buildings and infrastructures areas of biosystems engineering , Journal of Agricultural Engineering: Vol. 46 No. 1 (2015)
- Kamalesh Kanna S, Kumaraperumal Ramalingam, Pazhanivelan P, Jagadeeswaran R, Prabu P.C., YOLO deep learning algorithm for object detection in agriculture: a review , Journal of Agricultural Engineering: Vol. 55 No. 4 (2024)
You may also start an advanced similarity search for this article.