Leveraging deep semantic segmentation for assisted weed detection
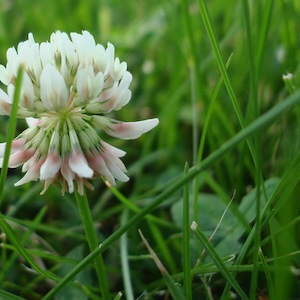
Published:18 February 2025
Abstract Views: 0
PDF_early view: 0
Publisher's note
All claims expressed in this article are solely those of the authors and do not necessarily represent those of their affiliated organizations, or those of the publisher, the editors and the reviewers. Any product that may be evaluated in this article or claim that may be made by its manufacturer is not guaranteed or endorsed by the publisher.
All claims expressed in this article are solely those of the authors and do not necessarily represent those of their affiliated organizations, or those of the publisher, the editors and the reviewers. Any product that may be evaluated in this article or claim that may be made by its manufacturer is not guaranteed or endorsed by the publisher.
Similar Articles
- Andrea Peruzzi, Luisa Martelloni, Christian Frasconi, Marco Fontanelli, Michel Pirchio, Michele Raffaelli, Machines for non-chemical intra-row weed control in narrow and wide-row crops: a review , Journal of Agricultural Engineering: Vol. 48 No. 2 (2017)
- Xiwang Du, Xia Li, Fangtao Duan, Jiawei Hua, Mengchao Hu, Static laser weeding system based on improved YOLOv8 and image fusion , Journal of Agricultural Engineering: Vol. 55 No. 4 (2024)
- Juquan Ruan, Shuo Liu, Wanjing Mao, Shan Zeng, Zhuoyi Zhang, Guangsun Yin, Fine-grained recognition algorithm of crop pests based on cross-layer bilinear aggregation and multi-task learning , Journal of Agricultural Engineering: Vol. 55 No. 3 (2024)
- Yun Zhu, Shuwen Liu, Xiaojun Wu, Lianfeng Gao, Youyun Xu, Multi-class segmentation of navel orange surface defects based on improved DeepLabv3+ , Journal of Agricultural Engineering: Vol. 55 No. 2 (2024)
- Kamalesh Kanna S, Kumaraperumal Ramalingam, Pazhanivelan P, Jagadeeswaran R, Prabu P.C., YOLO deep learning algorithm for object detection in agriculture: a review , Journal of Agricultural Engineering: Vol. 55 No. 4 (2024)
- Dhanashree Barbole, Parul M. Jadhav, Comparative analysis of 2D and 3D vineyard yield prediction system using artificial intelligence , Journal of Agricultural Engineering: Vol. 55 No. 1 (2024)
- Xiong Bi, Hongchun Wang, Double-branch deep convolutional neural network-based rice leaf diseases recognition and classification , Journal of Agricultural Engineering: Vol. 55 No. 1 (2024)
- Cesar Augusto Terán-Chaves, Sonia Mercedes Polo-Murcia, Cropping pattern simulation-optimization model for water use efficiency and economic return , Journal of Agricultural Engineering: Vol. 52 No. 4 (2021)
- Kai Tian, Jiefeng Zeng, Tianci Song, Zhuliu Li, Asenso Evans, Jiuhao Li, Tomato leaf diseases recognition based on deep convolutional neural networks , Journal of Agricultural Engineering: Vol. 54 No. 1 (2023)
- Bing Li, Jiyun Li, Key technology of crop precision sowing based on the vision principle , Journal of Agricultural Engineering: Vol. 54 No. 1 (2023)
You may also start an advanced similarity search for this article.